Symposium
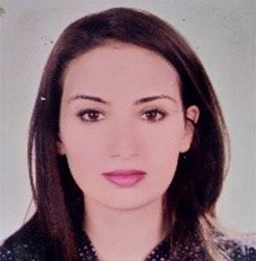
Symposium:
Proposal for Mini-Conference: Advances in Forecasting Techniques for Operations Research (AFTOR)
Dr. Marwa Hasni
LR-OASIS, National Engineering School of Tunis, University of Tunis El Manar, Tunisia
Dr. Marwa Hasni is an assistant professor in industrial engineering at the National Engineering School of Bizerte (ENIB). She holds a PhD from the National Engineering School of Tunis – University of Tunis El Manar and has been a member of the Laboratory for Optimization and Analysis of Industrial and Service Systems (LR-OASIS) since 2016. She also holds an engineering diploma in industrial engineering and logistics from the National Engineering School of Carthage – University of Carthage.
Her research focuses on the development and analysis of forecasting techniques applied to production systems, financial, and banking systems, with a particular interest in non-parametric sampling techniques, machine learning, and deep learning approaches.
She has submitted her accreditation to supervise research (HDR)
In addition to her research activities, Marwa Hasni regularly leads workshops and training sessions in the fields of artificial intelligence and business intelligence. She has also served as a panel chair in conferences and has been invited to speak at spring schools.
She has published and reviewed numerous research studies in leading international journals, including the International Journal of Production Economics, International Journal of Production Research, International Journal of Decision Sciences, Risk and Management, Managerial and Decision Economics, and the International Journal of Economics and Strategic Management of Business Processes.
Introduction
Forecasting plays a crucial role in operations research that enables organizations to anticipate demand, optimize resource allocation and enhance decision-making across various domains. The integration of advanced forecasting techniques, including machine learning and deep learning has significantly improved predictive accuracy and adaptability in uncertain environments.
This document presents the proposal for a mini-conference titled Advances in Forecasting Techniques for Operations Research (AFTOR), which will be organized as part of the ODSIE conference. The mini-conference aims to bring together researchers, practitioners, and industry experts to discuss recent developments in forecasting methodologies and their applications in operations research.
Proposed Professional Panels
- Machine Learning and AI-Based Forecasting for Operations Research a. Applications of machine learning and deep learning in demand forecasting
- AI-driven predictive models for supply chain optimization
- Case studies on AI-powered forecasting in industry
- Stochastic and Statistical Forecasting Methods in Decision-Making a. Time series analysis and probabilistic forecasting
- Bayesian and non-parametric methods for uncertainty modeling
- Hybrid approaches combining traditional and AI-driven techniques
- Real-World Applications of Forecasting in Industry and Services a. Forecasting in production planning, logistics, and healthcare operations
- Integration of forecasting models in decision support systems
- Challenges and best practices in implementing forecasting solutions